Statistics, as a universally acknowledged language of information, wields a forceful influence in our decision-making processes and perception of the world. However, the power vested in statistics can often be a double-edged sword as it presents its own set of complexities and potential pitfalls. The absence of a firm understanding of statistical intricacies and the context surrounding these figures can easily pave the way for negative ramifications, ranging from individual misconceptions to national policy errors. More importantly, the manipulation and misuse of statistics can immerse us in a whirlpool of confusion and misinformation, undermining the legitimacy, trust and clarity that statistics inherently offer. It is thus crucial to delve into the essence of statistics, the importance of contextual understanding, the potential for manipulation and misrepresentation, and finally, its real-world effects and consequences.
Understanding the Essence and Complexity of Statistics
The Statistical Misfire: The Perilous Consequence of Incomplete Understanding
In the vast expanse of scientific inquiry and research, the diligence of statistical reasoning stands as a cornerstone. A comprehensive understanding of statistics, harboring its subtleties and nuances, is an imperative facet of scholarly investigation. Ergo, a deficient comprehension or distortion of statistical principles can exacerbate both misinformation and misinterpretation, akin to a contagion proliferating through the cerebrum of the scientific community, and eventually to the public.
Wander through the realms of any scientific discipline, and the marker of statistics reverberates throughout. From discerning patterns in genomic data in the biological sciences, scrutinizing social trends in sociology, to the forecasting of economic tendencies in the financial world, predictive modeling and analysis relies heavily on sturdy statistical foundations. Thus, any whiff of negligence or deficiency in statistical competence can spur erroneous conclusions, leading to misinterpretation.
In scientific research, accuracy prevails; however, statistics is perceived as an ambiguous concept, often manipulated to serve specific ends. The tendency to cherry-pick data, present partial truths or merely the misinterpretation of statistical significance, when left unchecked, propels a discord in scientific validity. Consequently, this deficient comprehension nurtures a culture of misinformation, deteriorating the sanctity of the scientific method.
The popularization of the phrase ‘Correlation does not imply causation’ underscores the rampant misuse of statistics. Non-specialists, largely ignorant of the intricacies of statistics, may take correlational data at face value, confounding a mere association with a cause-effect relationship. This highlights the paramount need for enhanced statistical literacy, both within the scientific realm and among the public psyche.
The foggy understanding of p-values is another vulnerable spot. The ‘p-value’ — quite a misunderstood character in statistical calculations — is oftentimes misconstrued as a probability that the hypothesis is valid, which fuels flawed conclusions. In actuality, a p-value below a certain threshold merely suggests that statistically, the likelihood of the observed data given the null hypothesis is quite low. Hence, the intuitive leap to an inverse probability is a misstep — commonly made, yet treacherously misleading.
Furthermore, challenges lie in the realm of research replicability and the publishing bias towards positive results. A single study with statistically significant results may garner immediate attention, but if the findings are not easily reproducible, the initial results could very well be a false positive. Scientific rigor demands repeated verification, yet a preoccupation with positive results exacerbates our susceptibility to accept potential statistical aberrations as truth.
In essence, an incomplete grasp of statistical principles is a catalyst for misinformation and the spread of misleading interpretations. Given its perversion, those within the scientific and academic community must advocate for more stringent statistical evaluation and greater comprehensive understanding of statistical thinking. Only then can we hope to uphold the integrity of scholarly investigation, where the pursuit for truth isn’t muffled by the echoes of statistical misunderstanding.
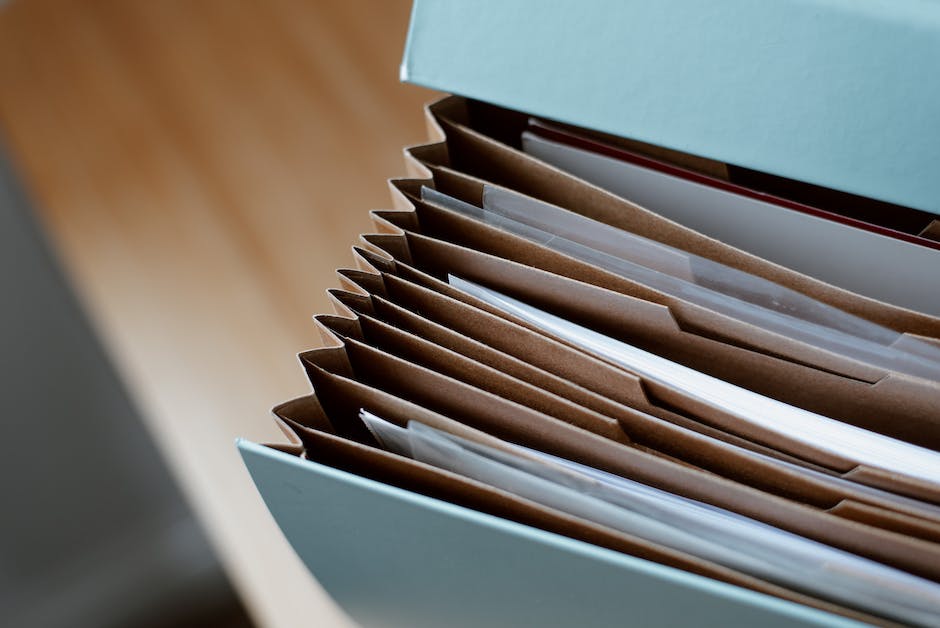
Contextual Understanding in Statistics
Exploring the Crucial Role of Context in Understanding and Interpreting Statistical Data
Empirical evidence and data, most importantly statistics, are like a lighthouse in the dense fog of scientific research. They guide us, they illuminate our path, they connect theories and perceived realities. However, navigating this data ocean is not without its challenges; the journey is nuanced, intricate, indeed fraught with risks of misinterpretation. One of the pivotal elements in accurate data interpretation resides in understanding the context.
Context, in relation to statistics, serves as the backdrop against which the numerical narrative unfolds. It offers a holistic understanding of the study’s conditions, underlying assumptions, as well as the applied methodology, informing us about the operational definitions and elaborate measurements involved. It is within this context that data assumes meaningful structure, transforming from haphazard figures into coherent information.
Considered metaphorically, statistical context breathes life into data. It sets the stage for the statistical story, deepening our understanding through finer details and shading. Without context, data is akin to bones devoid of flesh – stripped bare of its capacity to accurately reflect the nuanced complexities of reality.
For example, the declaration of a statistical figure of a 20% rise in rainfall only holds significance once the spatial and temporal criteria are made clear. Is this increase over a decade or a year, in a region experiencing drought or inundation, compared to normal rainfall amounts or anomalous periods? Contextual comprehension can make all the difference between significantly altering agricultural policies to slight adjustment of regional expectations.
Additionally, utilizing statistical data without the relevant context often leads to the ‘ecological fallacy’. This fallacy occurs when aggregate level data is used to make individual level inferences. The arresting relationships that manifest at the group level can often dissolve when inspected individually. This dissolution underscores the importance of understanding the broader implications of trying to map group level trends onto individual cases without the necessary contextual understanding.
Context also plays a vital role in determining the reliability and validity of statistical data. Without the inclusion of pertinent contextual details, we risk misinterpreting or misconstruing the data at hand. While a standalone number might seem impressive or damning, when placed in context, the narrative might change drastically.
It is crucial to note that the absence of context does not diminish the intrinsic value of raw statistical data. However, the derivation of accurate, responsible conclusions from the data palette necessitates the understanding and appreciation of its contextual artwork.
In conclusion, context serves as the foundation upon which statistical data unravels, providing the necessary blueprint for proper comprehension and interpretation. By underscoring the importance and indispensability of context in statistical interpretation, we seek to fortify the scientific endeavors and help ironsmiths of knowledge to accurately forge their respective contributions, driven by an in-depth understanding of the nuanced nature of statistical data.
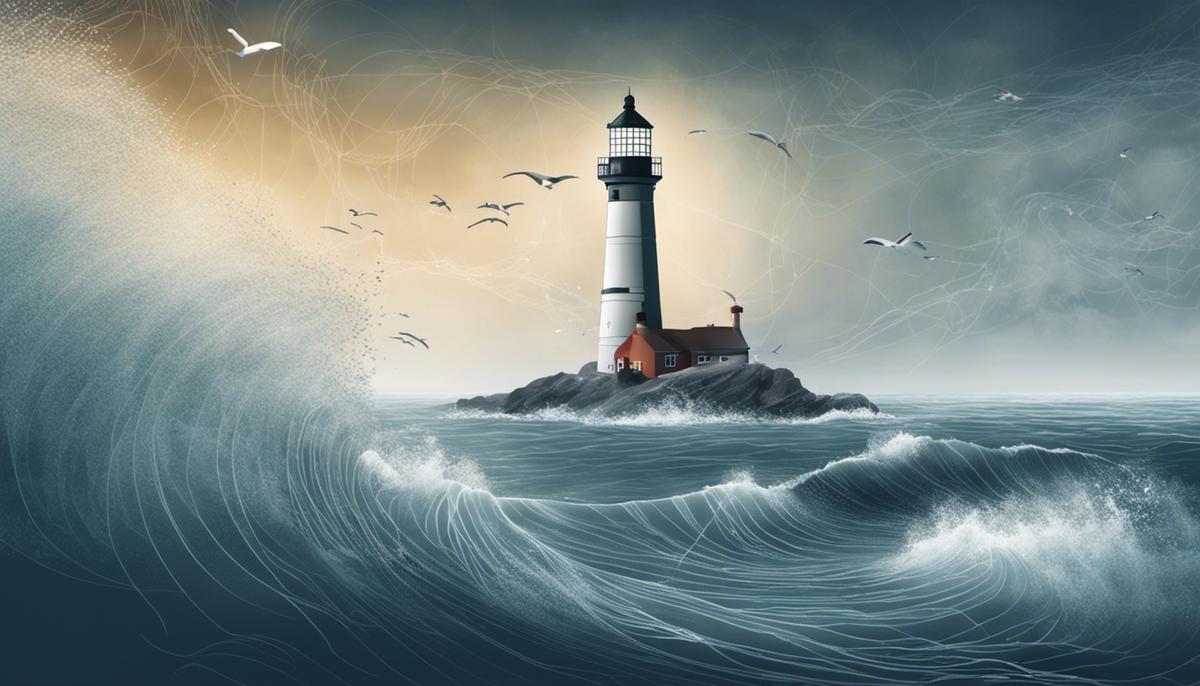
Manipulation and Misrepresentation of Statistics
Knowing the basic principles of statistics and understanding the essential role they play in scientific research and inquiry is not enough. An appreciation of context is equally vital in ensuring the correctness in interpreting statistical data. The role that context plays in statistics transcends the mere understanding of numbers and dives into the sociocultural, historical, political, environmental, or psychological backdrop that sets the stage for data creation and interpretation.
One remarkable illustration centers around the current situation that the world is grappling with – the global pandemic. Taking the case fatality rates of COVID-19, one might be quick to compare the numbers between two countries purely based on these figures. However, without the appropriate context – such as differences in population size, health care access, testing rates, and demographics, among others – such comparison is not only flawed but can also potentially lead to far-reaching and unfortunate implications.
This example emphasizes that data interpretation is as much about knowing what’s not said, as it is about understanding the figures presented. One should also consider studies’ design, experimental conditions, selected variables, and even biases inherent in the researchers or the subjects. Overlooking these contextual factors amounts to surrendering to the ecological fallacy, where one incorrectly deduces conclusions about individual-level behavior from group-level data.
Notably, the ecological fallacy, while seemingly simple, has led to notorious misinterpretations in statistical history. The flawed assumption that the behavior of an individual can be accurately predicted from the averages of a group can lead to erroneous data analyses. This consequence acts as a stark reminder that understanding the trajectory from the individual to the aggregate is one of the complex yet crucial aspects in statistics.
Diving deeper into this intricate connection between statistics and context, one can’t help but acknowledge the difference between raw statistical data and contextualized data interpretation. Raw data, stripped of context, may appear abstract and meaningless. Contextualized data, however, tells a story which directly influences the interpretation and conclusion that one arrives at. Without the right context, data might be misused or manipulated, whether intentionally or unintentionally, losing the true essence of the findings.
This point steers toward the crux of the matter that even the most accurate and well-meaning statistical results can be dangerously misconstrued or misrepresented, due to various reasons – from the simple lack of knowledge to more malicious motivations. A diligent effort, thus, must be taken to not only distribute numerical literacy and understanding among researchers and the public but also to place due emphasis on context.
Ultimately, the efficacy of statistical results in the creation of knowledge and research largely depends not only on the mathematical operations performed on the data but also on how painstakingly the contextual factors have been considered. This consideration embodies the art of statistical analysis, transcending the realm of numbers and seeking evidence in the corners of its own context to create an accurate narrative.
Interpreting statistics without context is akin to reading a book with every other page ripped out. The essence is lost, the narrative is disjointed, and the story is essentially incomplete or even inaccurate. Hence, it can’t be stressed enough: context is key. And it is this key that will unlock the true power of statistics in paving the path to scientific discoveries and growth.
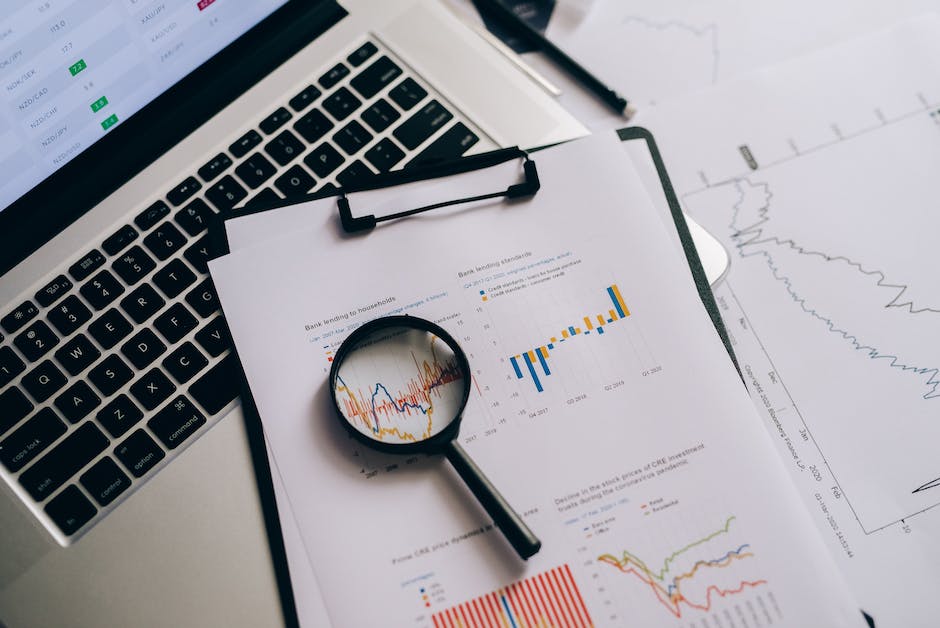
Real-world Effects and Consequences
The potential damage and consequences of decontextualized statistics range from misinformed policy measures to potentially catastrophic societal actions, the dangers of which cannot be understated. A critical aspect of comprehending statistics lies not only in the study of data but also the circumstances in which they are derived, or their context. When this context departs, a variety of potential risks emerge. This occurrence, dubbed as ‘decontextualization’, might undermine the credibility, reliability, and inherent truth these figures designate.
The most profound danger of decontextualized statistics is the proclivity to misinform, creating a distorted perspective of the situation at hand. The absence of context obscures significant inherent nuances – an over-simplification that reshapes truth. For instance, comparing GDPs across nations without prosecuting factors such as purchasing power parity and per capita income could engender unjust economic policies, contributing to growing disparities.
Moreover, decontextualized statistics might inadvertently bolster stereotypes and promulgate misinformation. In utilizing figures without the cloak of conditions from which they were instigated, certain groups may be unjustifiably pigeonholed or discriminated against. An example of this can be seen in the interpretation of crime statistics which, devoid of the crucial socio-economic factors, can lead to erroneous assumptions about certain demographic groups.
A particularly alarming repercussion of decontextualized data is observed in policy-making decisions. The dire consequences of basing decisions on decontextualized data cannot be overstressed in the realms of healthcare, economics and social welfare where understanding the macrocosmic picture is fundamental. Policies foundamented on statistics stripped bare of context may lead to ineffective or even detrimental initiatives, wasting valuable resources and potentially causing harm.
Additionally, decontextualized data can compromise the comparative potential of statistics. A blatant incongruity emerges when attempting to compare numbers generated from dissimilar contexts. Therefore, discourse based on this comparison may lead to erroneous conclusions.
Context serves beyond merely a backdrop to data. Instead, it shapes the intrinsic meaning of numbers, conflating the stark black and white figures with shades of grey complexities. Without it, statistical figures may become sturdy tools for manipulation in the hands of those seeking to twist truth for their personal narrative.
Therefore, in comprehending and deploying statistics, it becomes crucial to prod, not just the data, but also their origins, the circumambient context. To prevent the exploitation of data, decontextualized numbers must be treated with discretion and an insistent curiosity for their source. Achieving this necessitates a concerted effort to bolster statistical literacy. It forms the bedrock of robust scientific communication, public engagement with scientific discourse and facilitates the delivery of reliable statistics purported to inform policies and transform lives.
In this scientific endeavor, understanding that figures carry no inherent meaning, but rather, meaning ordained by their context, is fundamentally important. Recognizing the depth of this truth leads one towards a more informed, objective, and humble approach to scientific research and related fields. Unleashing the power of numbers is no small feat, and in doing so, the preservation and acknowledgement of their contextual lineage should unfailingly remain central.
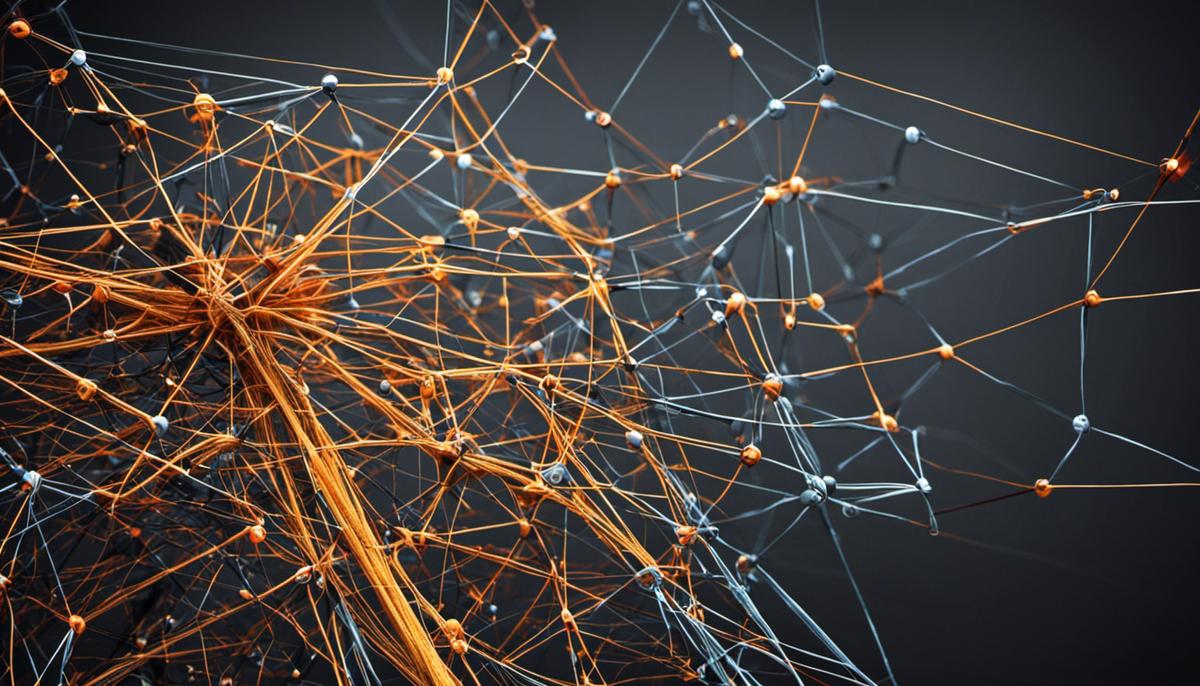
Scrutinizing several instances where inaccurately construed statistics resulted in damaging consequences has highlighted the grave imperative of a comprehensive understanding of this complex field. Learning from these historical and contemporary cases, we should collectively advocate for and practice statistical literacy, thus fostering a more informed and discerning public sphere. When statistics are correctly understood, analyzed and presented, these powerful tools can provide invaluable insights and contribute substantially to fields ranging from public health to economics, and beyond. Therefore, the onus is on us as a society to grasp the underpinnings and appropriate use of statistics, in order to prevent manipulation, promote truth, and progress with accurate knowledge.
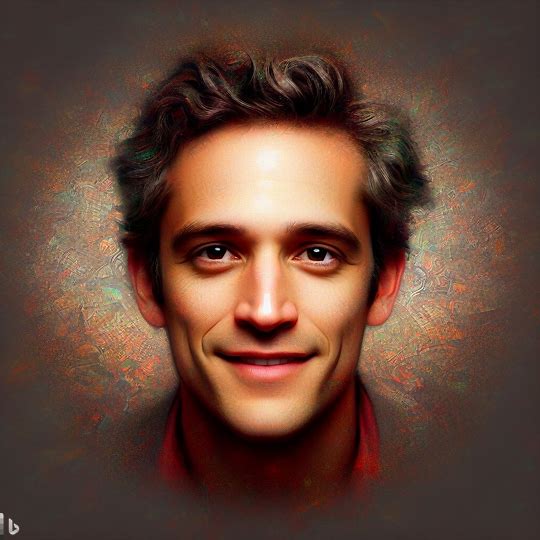
Matt Smith is a seasoned journalist and author whose expertise spans across the dynamic realms of Politics, Gadgets, Gaming, and a plethora of general interest topics. With a Master’s in Political science and tech pedigree shaped in Silicon Valley, Matt brings a wealth of knowledge and a critical eye to everything he writes.
Politics: Matt offers sharp political commentary, drawing from his experience as a political analyst and his academic rigor.
Gadgets: His tech insights are grounded in real-world experience, having been on the front lines of innovation with a degree from Caltech.
Gaming: A respected voice in gaming, Matt’s reviews and trend analyses are a testament to his deep involvement in the gaming community.
General Topics: From science to culture, Matt’s writing spans a broad spectrum, engaging readers with a blend of expertise and relatable prose.
Engage with Matt’s compelling content for a fresh perspective on the issues at the forefront of today’s discourse.