Within the expansive realm of data analysis, statistics play a pivotal role as they quantitatively derive insights from raw data, facilitating both understanding and decision-making processes. However, like any tool, they’re not impervious to manipulation, misinterpretation, or bias, frequently skewing our perception of reality. This paper delves into the intricate nature of statistical bias, spinning a narrative that traverses through the inherent variability of statistics, the consequences of poor sample selection, and the issues that arise from oversimplification. It illuminates the under-discussed practice of cherry-picking statistical data, dissecting how this artful dance of selective representation can profoundly alter perceptions. Furthermore, tackling the psychological element of confirmation bias, the scourge of objective understanding, it illustrates how our predisposed beliefs can subconsciously influence our interpretation of statistical data.
Table of Contents
The nature of statistics and their susceptibility to bias
The Innate Bias in Statistics: An Examination
Statistics, as a discipline, exists at the nexus of mathematics and sociology, the overlapping areas where numbers meet people, where raw data meet human woes and wonders. However, it would be an egregious oversight if we failed to address that this magnificent field, given its interconnected and intricate nature, has an inherent tendency toward bias. Deeply rooted in the processes of statistical design, analysis, and interpretation, this bias can influence outcomes, and consequently, crucial decisions based on these statistical results.
Let’s begin with the design, the germinating point where the seeds of bias could potentially be sown. Each study or experiment commences with a question; however, the research question’s phrasing can significantly modulate the results. If a question is formulated with a preconceived notion or expectation, it can subsequently guide the design, veering it towards a specific answer – a phenomenon often termed “confirmation bias”.
Sampling methods further propagate this inherent bias. A truly random sample is hard to achieve, and often, the set of data collected is skewed towards some particular characteristic. This phenomenon, known as ‘selection bias’, can usher in erroneous results and conclusions. Moreover, the data collected may inherently reflect pre-existing societal biases, amplifying them in the statistical analysis – a predicament termed ‘algorithmic bias’.
Then, we pivot to statistical analysis. Implicit bias can surface in the selection of statistical tools or models applied. Suppose a researcher chooses to use a linear regression model when the data do not meet the necessary assumptions. The final results, then, could be heavily biased, exuding significant implications for studies leading to policy decisions or medical treatments.
But even the most meticulously designed and conducted studies can be led astray in the final phase: interpretation. Regardless of the objectivity of numerical results, the interpretation process inherently bears a human touch, and an inadvertent personal or cultural bias could transform the results. For instance, a researcher might lean towards ‘overfitting’, whereby the analysis tightly fits the data at hand but fails to generalize other data, resulting in the ‘overfitting bias’.
Throughout all these phases, the potential for bias remains lurking, poised to strike and skew results. But it’s fundamental to remember that recognizing these fallacies is not a testament to the failure of statistics. On the contrary, it’s a necessary step in our collective quest for improvement.
Awareness and conscientious attention to these innate biases in statistical processes is our first line of defense. Ongoing research in bias mitigation is dedicated to developing methodologies that help counterbalance these biases, ensuring that statistics continue to serve as an invaluable tool in our perpetual endeavor for knowledge and scientific advancement.
As we grapple with these complexities, let’s uphold the belief that our continued scrutiny will allow statistics to better reflect the intricate tapestry of our world, bias-free. Indeed, our passion for this realm encourages us to take these challenges head on, driving us further into our journey through the fascinating world of statistics.
Remember, in the immortal words of George E.P. Box, “All models are wrong, but some are useful.”
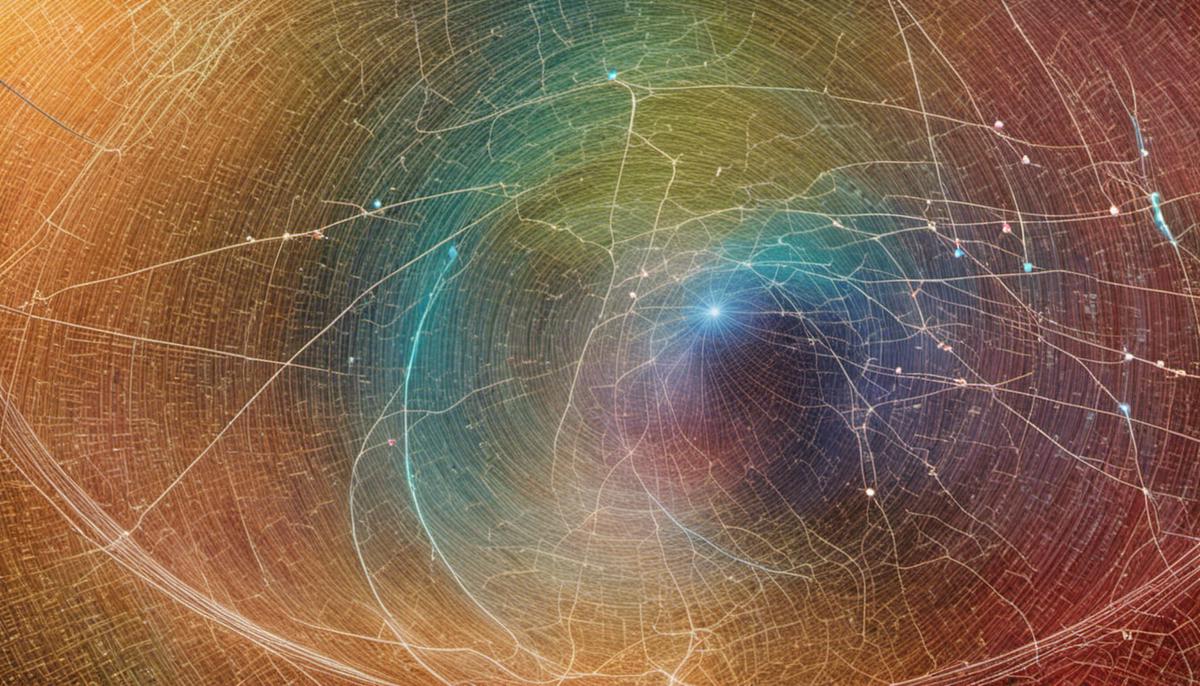
The role of cherry-picked data in skewing perceptions
Delving Deeper: Cherry-Picking Data and Its Contribution to Statistical Bias
Statistics arguably represents the confluence between empirical evidence and knowledge, forming the backbone of myriad scientific advancements. Yet the field is not immune to certain malpractices, among which, cherry-picking data poses a pertinent concern. As with every facet of statistical processes already elucidated upon, there lies an undercurrent of bias in this aspect as well. This practice veers dangerously close to the human proclivity for affirmation rather than information, distorting our understanding of issues at hand, and consequently, the scientific advancements predicated on these statistics.
The act of cherry-picking data manipulates evidence—an action that is antithetical to the true essence of statistics. In this practice, typically, only subsets of data that fulfill predetermined criteria, often biased, are employed. Such selective use invariably skews the representative power of the entire data-set, thus inducing an element of bias in the statistical analysis. This ultimately lends credence to misleading narratives, deviating from the primary motive of furthering genuine knowledge and research.
In its more subtle form, cherry-picking often disguises itself through a rigorous search for patterns. Habitually, multiple models are fitted to a data-set, and the ‘best’ results are emphasized upon at the expense of others, thus violating the principle of rational, all-encompassing analysis. Further exacerbating this issue is the propensity for these biased ‘results’ to get propagated and amplified in academic and media channels, thereby leading to a grossly distorted understanding of the issue at hand.
At its core, cherry-picking data not only devalues the integrity of statistics but also stands in complete contradiction to the field’s inherent intricacy and dynamism. A rigid, predetermined notion lessens the possibility of extracting unanticipated insights from data, thereby stifling the spirit of scientific curiosity.
Despite the grim scenario, it is essential to perceive this as a call for greater vigilance and adherence to rigorous statistical protocols. Regular recalibration of research methodologies to avoid data cherry-picking, stringent peer-review processes that cast a critical eye on data selection, along with increased transparency in data collection and processing methods, are potential ways forward.
In conclusion, George E.P. Box’s wise words continue to resonate in the realm of statistics, “All models are wrong, but some are useful.” While learning and adapting to make them more useful, the community should remain conscious of the risk of biases. Acknowledging these risks, cherishing the complexity of our statistical tools, and constantly optimizing for accuracy is an homage to this intricate, informative, and immensely powerful field.
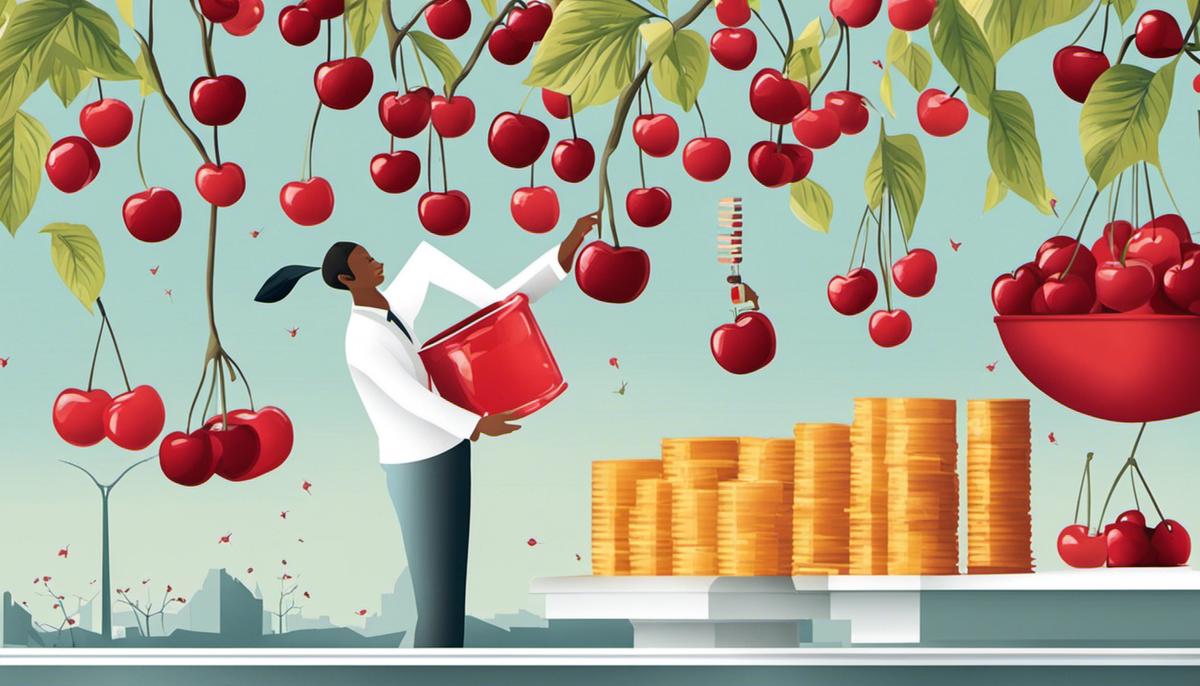
Confirmation bias and the misuse of statistical data
In the ongoing pursuit of understanding the world through a scientific lens, the tool of statistics plays an indelible role. However, its power lies not merely in the assemblage and analysis of numbers, but rather its conscientious application, which can often be compromised by what is termed as ‘cherry-picking data.’
Cherry-picking data, a form of confirmation bias, refers to the selective assembly of statistics and data that supports one’s preconceived notions or hypotheses while conveniently overlooking data that contests the same. A venerable pitfall to the sanctity of research, it can lead to the deliberate distortion of statistical scrutiny to favor certain outcomes.
The manipulation of evidence through cherry-picking data hits upon the very crux of scientific investigations by mitigating the objectivity, thereby obscuring our understanding. Presenting a skewed vision of the subject under scrutiny, it strengthens the grip of conformation bias, empowering the default human inclination to seek evidence in compliance with their beliefs, making them more prone to embracing distorted results.
The subtle play of cherry-picking data can also manifest in emphasizing selected results that align with the sought-after deduction and projecting patterns that are not representative of the comprehensive dataset. These skewed representations pervade academic publications and media channels alike, fostering a distorted understanding among the masses.
Such practices stand in stark contrast to the guiding principles of statistics: integrity and honest representation of data. While they might advance short-term objectives, they essentially compromise the long-lasting pursuit for truth and understanding. Consequently, a disregard for the foundations of statistical practice fosters an ecosystem of disillusionment and misinformation.
The road ahead calls for a profound commitment to maintaining the integrity of statistical investigations. A rigorous adoption of statistical protocols, including calculated and unbiased data collection, can significantly reduce the malpractice of cherry-picking data. Furthermore, peer-review processes appointed with examining the methodology of statistical research can further consciousness and counter confirmation bias.
In concert with these measures, transparency in data collection and processing can serve as a crucial deterrent against biased practices. The open sharing of data and methodologies can allow for reproducibility, the cornerstone of scientific credibility, fostering an environment of shared knowledge and progress.
At its heart, the field of statistics carries the potential for tremendous consequences, both advancing and detrimentally impacting our understanding of social and natural phenomena. Its power is both complex and potent, urging the need for the continual refinement in its practices. As we harness this tool, it’s pivotal to remember that statistical accuracy stems from the rejection of confirmation biases, thereby echoing George E.P. Box’s assertion – while all models are flawed, some are useful. Maintaining this perspective furthers a commitment to seek truth, and in doing so, advance scientific knowledge.
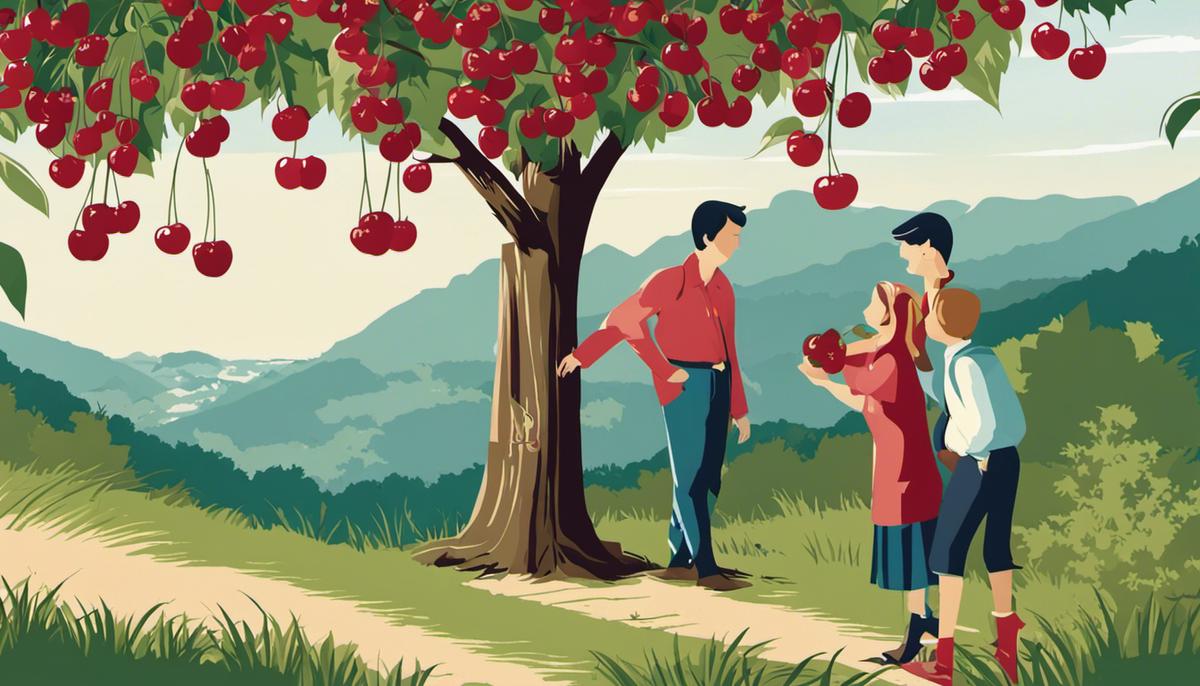
Case studies of statistical bias in real-world situations
Deciphering the world through a scientific lens requisite the inviolable technique of statistics. A pervasive, yet frequently unrecognized bias at play in both data collection and analysis is the propensity towards cherry-picking data.
This encompasses selecting subsets of evidence or statistical results that magnify distinct patterns and disregard inconsistent data. Effectively, it’s a form of confirmation bias – yielding a skewed depiction that supports a desired narrative. This introduces the precarious task of distorting statistical examination and manipulating evidence.
The implications of cherry-picking data extend far beyond merely inaccurate analysis. There’s a seismic disruption in our comprehension of phenomena, clouding the link shared by data and evidence. When we permit the erosion of statistical scrutiny’s authenticity, we augment the risk of propounding flawed or misleading assumptions.
Biased results that stem from cherry-picking data can proliferate through academic discourse and media channels. There’s a propagation of distorted comprehension of the issue at hand. This can generate misguided perceptions and spur erroneous policymaking, thereby underscoring the necessity for undistorted representation of data in the statistical landscape.
Adherence to the principles of statistical integrity is thus not merely an academic necessity but a societal obligation. Ensuring an unbiased comprehension of data is instrumental to maintaining the integrity of statistical investigations.
Championing the need for well-established statistical protocols, comprehensive transparency, and unbiased data collection is pivotal to rectify this. Moreover, researchers should actively seek to countermand potential biases, recognizing the inherent propensity towards such distortions.
Peer-review processes can offer significant counterweights to such confirmation biases. By enforcing stringent review mechanisms, we can scrutinize the methodology holistically, illuminating the potential discrepancies and biases. Additionally, an emphasis on transparency in data collection, interpretation, and processing can circumvent cherry-picking and ensure the data’s authenticity.
Furthermore, a meticulous statistical practice perceives the brandished numerical data not as mere figures but dialogue between social and natural phenomena. Therefore, we must constantly refine our methods, seeking precise, unbiased interpretations.
Above all, it is incumbent upon us to reject any inclination towards confirmation biases, fostering a culture that aspires for empirical truth. To fully harness statistical potential as a powerful tool of understanding, it’s time to renew commitment towards statistical credence while eradicating sources of bias. Only then will the statistical data transform from mere figures into valuable scientific knowledge.
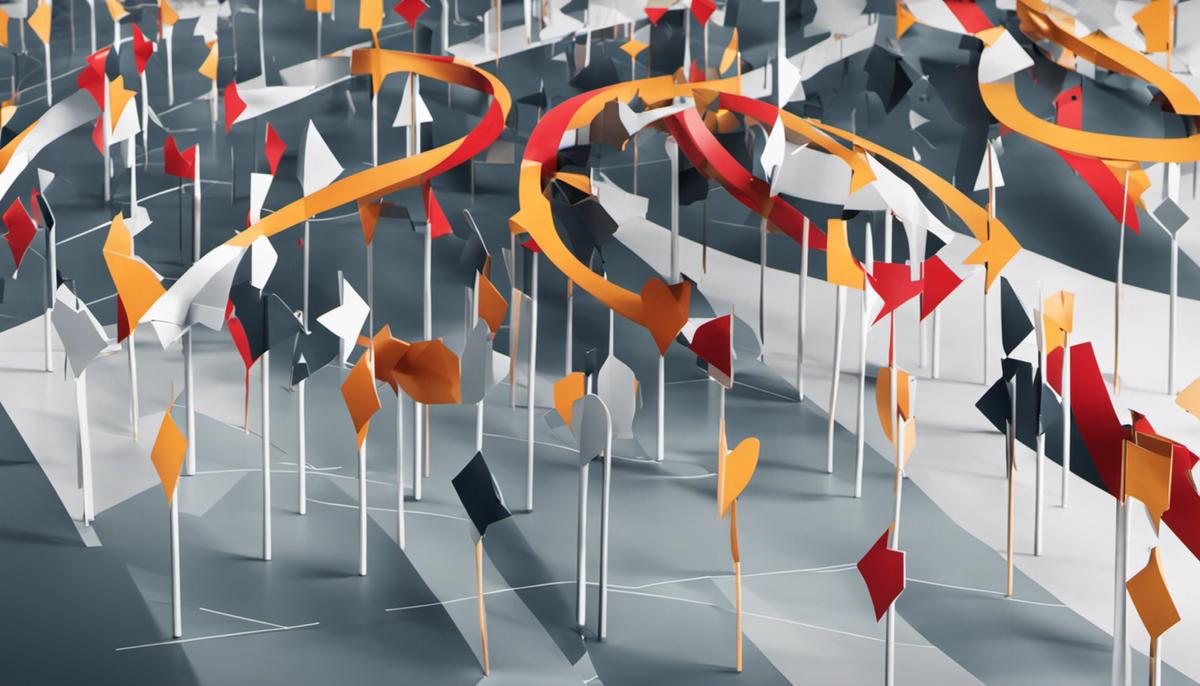
Stepping back, it is evident that albeit statistics’ undeniable power, we must approach them with informed caution. As this paper has shown through an array of theoretic discussions and real-world case studies, statistical data can easily fall prey to biases, be it through cherry-picking, confirmation bias or simply poor sample selection. Ultimately, these biases can have profound effects on our understanding, influencing our perception of various crucial issues in areas such as healthcare, economics, and social science. Therefore, it is imperative that we nurture our ability to discern both the explicit and implicit biases in statistical data, fostering a more informed, objective understanding of the world around us.
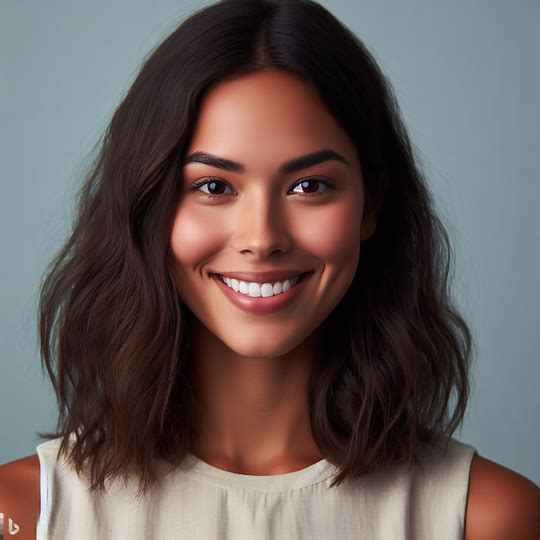
Amara Rainforest is an authoritative voice in business analysis, blending her MBA in Finance insights with real-world consulting experience. She distills complex market trends into clear, actionable advice for her readers. Her engaging writing captures the essence of modern business challenges and triumphs, making her a must-follow for aspiring entrepreneurs and seasoned executives alike.