In an era where decisions are often driven by data, understanding the nature of raw data becomes vitally essential. Raw data, the unprocessed direct-from-source information, serves as the backbone of accurate studies and reports. However, even the most credible data set can lead to egregious errors if not correctly interpreted. This reality reflects the dire need for people to comprehend the stark difference between raw and processed data and how the alteration of data from its pure form can dramatically impact results. Furthermore, the episode of common errors in statistical analysis, selection bias, and other factors that lead to misinterpretation expose a worrying predicament that potentially skews scientific conclusions and misdirects public opinion.
Table of Contents
Understanding the Nature of Raw Data
The Intrinsic Value and Distinctiveness of Raw Data Versus Processed Data
In our data-driven society, the importance of raw data and processed data cannot be overstated. However, it is crucial to understand their distinctive characteristics, underlying peculiarities, and the different roles they play in the realm of research and knowledge acquisition. This article endeavors to elucidate those differences, exploring the intrinsic worth and uniqueness of either type, and will demystify the concept of raw data as juxtaposed with processed data.
Raw data stands as the preliminary, unaltered version of data that originates from the source. It is in an unprocessed form, retaining every minute detail of information as directly gathered. This makes raw data a veritable gold mine of crucial facts, untarnished by subjective influence or interpretation.
Indeed, the power of raw data lies in its untouched and comprehensive state; this attribute segues into one significant advantage – versatility. Raw data can be utilized across myriad research purposes due to its broad range of context and details. This wealth of information also permits in-depth explorations into different aspects of the same topic, unveiling nuanced perspectives not always apparent in an initial observation.
Simultaneously, raw data presents itself in a more challenging format to comprehend. It often takes shape in an unstructured format, necessitating higher analytical skill sets and advanced tools for transformation into meaningful information.
On the other hand, processed data emerges as a polished and tailored version of raw data. It is data refined through processes such as aggregation, organization, and interpretation. As such, processed data is streamlined and presents information in an easily digestible format, making it more accessible to source meanings, draw conclusions and make definitive decisions.
It’s worth noting that the processing of raw data into a more curated manifestation necessitates a degree of interpretation. Consequently, it may lose some granularity of detail present in raw data, and the risk of skewing data towards a particular perspective remains, depending on the methodology used for processing.
Furthermore, the reduction in complexity and higher understandability makes processed data more suited for decision-making processes, modeling, predictions, and presentations. Its tailored synthesis presents insights in ways non-experts can grasp, aiding in comprehensive dissemination of information.
In conclusion, raw data and processed data both hold distinctive sets of advantages and limitations. Raw data’s uniqueness lies in its unprocessed state, offering a more granular, untouched, and versatile source of information. Processed data, however, with its refined structure and ease of comprehension, presents an accessible insight platform suitable for decision-making and knowledge dissemination.
Therefore, the dichotomy exists not in the value of either type over the other, but in understanding their different roles and leveraging them appropriately within various applications. After all, it is the judicious and effective usage of data, in its many forms, that raises the curtains on new comprehensions and knowledge.
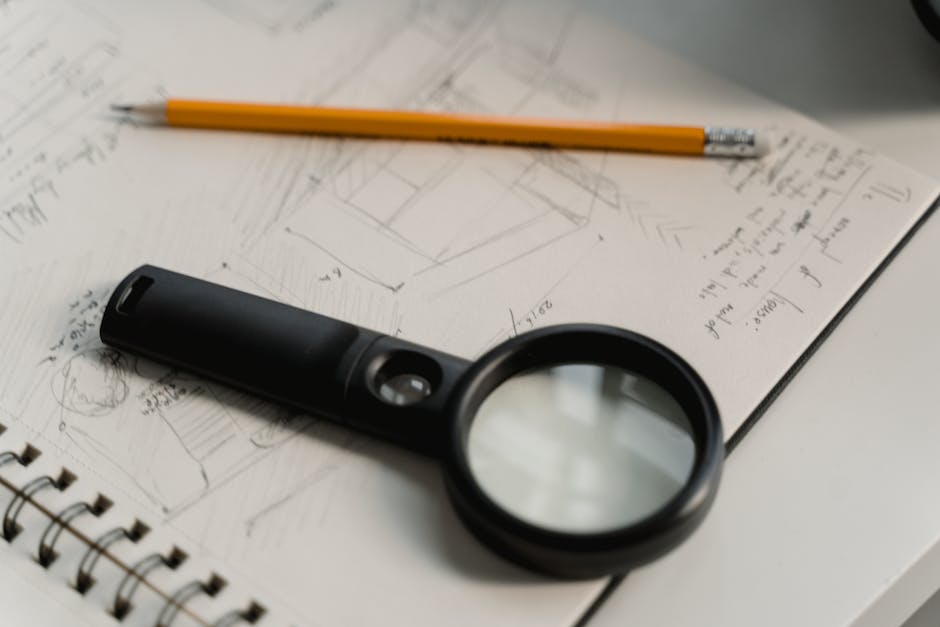
Common Misinterpretations of Raw Data
The Interpretation of Raw Data: Common Errors and Pitfalls
After establishing a firm understanding of raw data and its role in furthering knowledge and research, the critical essence to be addressed is how to interpret this data accurately. There are countless opportunities for misinterpretation and error here, as raw data is inherently complex. While the abundance and depth of the information it provides initially seem inviting, addressing these complexities correctly is paramount to gaining accurate insights. This article will delve into some of the most typical errors researchers unwittingly fall prey to during the interpretation of raw data.
One such common pitfall is utilizing inappropriate analytical methods. This is where the unstructured and complex nature of raw data can pose a challenge. It is crucial for researchers to employ the proper statistical tests and analyses that suit the data at hand. This choice is influenced by multiple factors, such as the data’s nature, the type and number of variables involved, and the purpose of the conducted research. The wrong choice of analytic tactics can lead to grossly inaccurate interpretations and findings.
Another frequent mistake is neglecting missing or ambiguous data points. It is expected to encounter some data that is unclear or incomplete when dealing with raw data. Instead of discarding these pieces, inadequate handling or replacement could distort the end results. It is essential to tackle missing or ambiguous information systematically and transparently to prevent introducing bias to the findings.
A third common error in raw data interpretation is assuming causation from correlation. While two variables might be seen to move together, this does not necessarily mean that one causes the other. This assumption can lead to spurious conclusions and misinformation. To ensure robust interpretations, it is necessary to consider other potential variables that may be influencing the correlation and to use controlled experiments where viable.
Drawn into the allure of finding patterns, researchers sometimes fall into the trap of overfitting their models. Overfitting occurs when a statistical model is so closely tailored to the existing dataset that it loses predictive power for new data. The model effectively memorizes noise from the raw data instead of learning the actual underlying patterns. Avoiding overfitting involves using methods such as cross-validation and regularisation, which help ensure the model’s performance on unseen data.
The fifth common error refers to disregarding potential bias sources in the data. Raw data collected can often include systematic biases, some of which may be subtle and unknown. These biases may creep into the data via flawed data collection methods, selective data inclusion, or even through the algorithms that process this data later. Unaddressed, these biases can significantly skew results and lead to faulty conclusions.
In closing, a nuanced understanding, and cautious interpretation of raw data are crucial for the progression of any research or investigation. Avoiding common errors requires methodical analysis, judicious employment of statistical methods, and meticulous bias examination. Such vigilance ensures the credibility and reliability of findings, thereby reinforcing the structure of knowledge built on this robust foundation. By arming themselves with this knowledge, researchers can tread the potentially treacherous path of raw data interpretation with a higher degree of confidence and accuracy. Let this work serve as a testament to a broader appreciation of the role, challenges, and excitement packed in the realm of raw data interpretation.
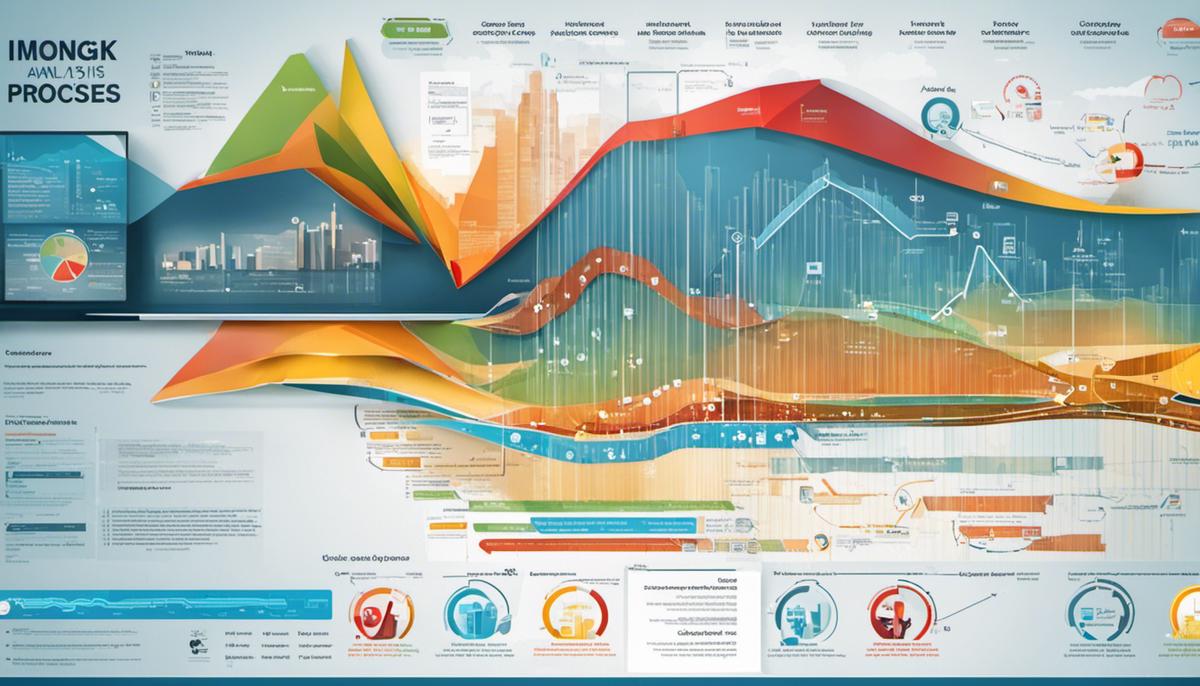
Impact of Raw Data Misinterpretation
The Misinterpretation of Raw Data: Implications for Research and Applications
Within the complex labyrinth of scientific research, the interpretation of raw data is a crucial but intricate process. It demands adept understanding and careful application of analysis to effectively convert this unprocessed information into useful knowledge. Regrettably, the intricacy of this process opens doors for data misinterpretation, consequently impacting the research outcomes and their applicability. This section will shed light on a variety of issues that arise from the misinterpretation of raw data.
When the analytical approaches applied to raw data are inappropriate, unsound conclusions are often drawn. Embarking on the analytical journey with incorrect statistical techniques can distort the patterns and relationships hidden within the data. For example, if complex data with multiple interacting variables is erroneously analyzed using a simple linear relationship, the results become skewed and misleading. This erroneous application could spawn findings that are drastically divergent from the actual data trends.
Handling missing or ambiguous data points incorrectly can also be a source of data misinterpretation. Each data point within a dataset contributes to the overall narrative that the data is detailing. When these missing or ambiguous data points are improperly managed — by ignoring them, or worse, imputing values without a robust method — the resulting analysis can be erroneous and misguide the research findings.
Recognizing the difference between correlation and causation is another critical aspect of data interpretation. Detecting a correlation, or a mutual relationship between two variables, does not necessarily imply a causal link. It has been too often noted in research that a correlation in data is rashly interpreted as causation, leading to ungrounded conclusions and theories. This is a scientific fallacy that has far-reaching implications for the validity of research findings and their subsequent applications.
Overfitting statistical models constitutes another potential pitfall in the interpretation of raw data. This occurs when a model is tailored to fit the training data too closely, losing its predictive power for new data. Overfitting contributes to results that are impressively accurate in the initial dataset but fail to extend their accuracy to further data. This can mislead researchers into believing relationships exist in their data when they do not, resulting in flawed conclusions and applications.
Lasty, the issue of bias is a constant specter in data interpretation. Failing to identify potential sources of bias in data leads to skewed results and misinformed decision-making. Researchers must vigilantly check for these potential imperfections by scrutinizing experimental designs and database characteristics, among other factors.
In conclusion, misinterpretation of raw data can occur in a multitude of ways, each having profound implications on the research outcomes, and their applications. An understanding of these potential misinterpretations is integral to fortify the robustness of scientific findings and, ultimately, propel the vehicle of scientific progress forward with integrity.
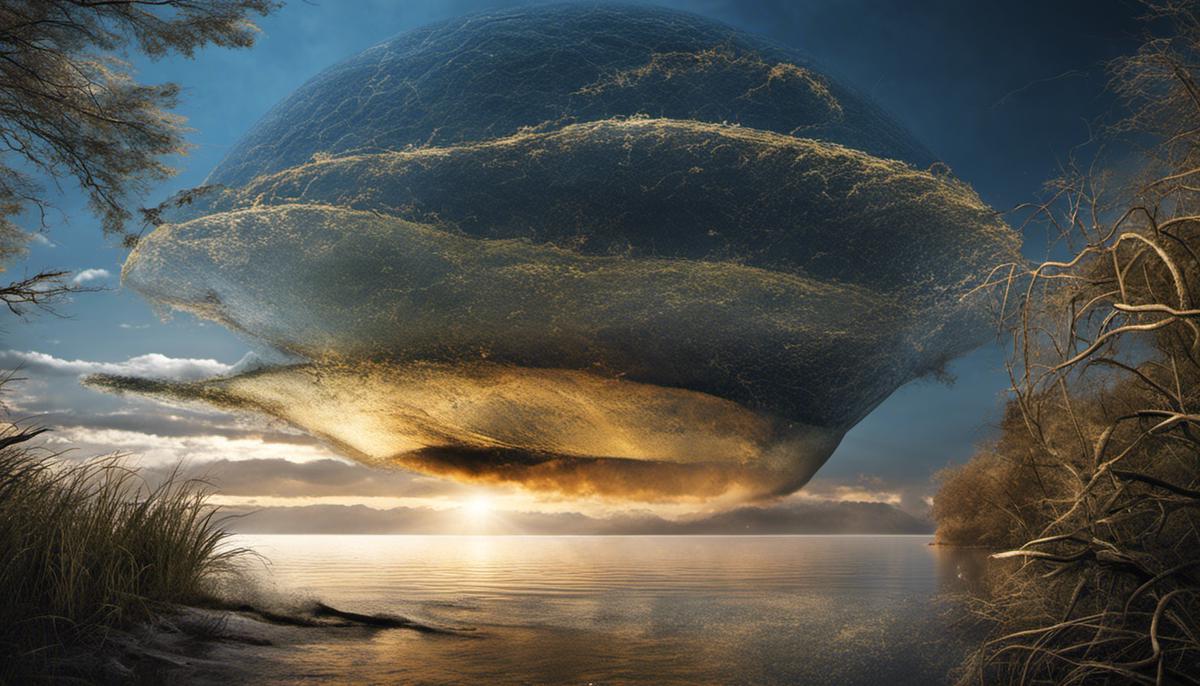
Preventing Data Misinterpretation in Science
Maintaining fidelity to the integrity and implications of raw data demands the development of rigorous and systematic procedures. It is essential to adopt best practices that not only help in avoiding misinterpretation but also enhance the reliability and validity of research outcomes.
The first step towards such achievement converges on the establishing of clearly defined objectives and relevant indicators. Data should always be contextualized within the framework of distinct aims and expectations. This allows one to filter out unnecessary data and lessens the risk of erroneous interpretations induced by irrelevant data points.
Equally integral is the application of suitable analytical methods congruous with the nature and scale measurements of the data at disposal. The indiscriminate application of statistical tests can lead to invalid results and misleading conclusions. A comprehensive understanding of the principles of statistical analysis is imperative for effective data interpretation.
Ensuring robustness in data analysis also emphasizes the importance of being cautious about missing or ambiguous data. Data scientists should not assume that such patterns are randomly distributed as they may lead to systemic biases. Strategies like multiple imputation, that create plausible values for missing data, may assist in addressing this issue.
A common pitfall in data analysis is the mistake of interpreting correlation as causation. Correlation merely implies a relationship between two variables, not that one causes the other. It is therefore crucial to remain critically skeptical and formulate hypotheses based on theoretical understandings, not just observed associations.
While achieving a good model fit is desirable, one must beware of the tendency to overfit models. Overfitting occurs when a model fits the data too well and captures every minor fluctuation, including the noise. Such models could provide poor results when confronted with new data. Bias-variance tradeoff, that seeks to balance complexity against generalizability, is a concept that researchers can employ to deal with this issue.
Last but certainly not least, recognizing and accounting for potential biases is of essence. Bias, the systematic deviation from the truth, can infiltrate at every stage of research, from data collection to interpretation. It is vital thus to scrutinize the research design and analysis for any potential sources of bias, and adjust for or, minimize their impact, for instance, through techniques like stratification or propensity scoring.
The underpinning of the above practices is a commitment to continuous learning, a blend of skepticism, curiosity and humility, and an unerring devotion to the principles of scientific integrity. Remember, data reciprocates the respect it is treated with, thereby illuminating a path to truth and discovery.
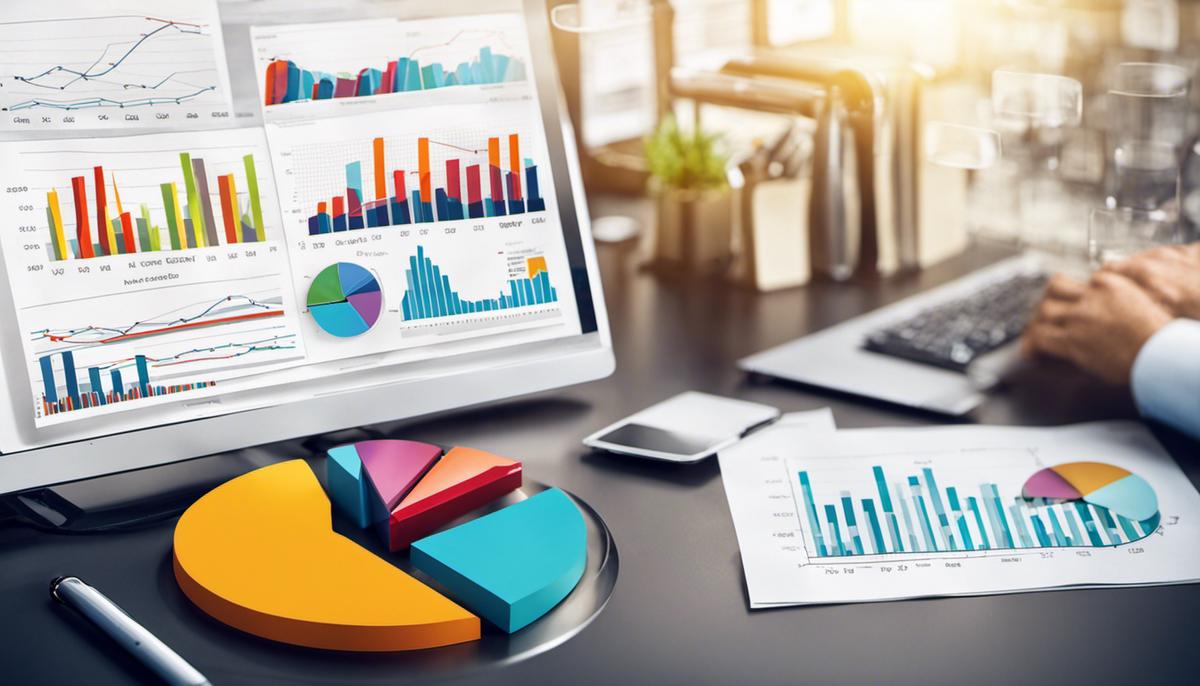
The challenges born out of misinterpretation of raw data are serious, but by applying a stringent adherence to ethical guidelines in data handling, meticulous data management, appropriate statistical methodology and rigorous peer-review processes, they are not insurmountable. Highlighting these preventive measures not just informs those involved in the data interpretation process but also serves as a clarion call to users of interpreted data to scrutinize the bases of their data-driven decisions. As we continue to rely heavily on data to shape our world, it becomes an essential task for all to ensure the accuracy and reliability of this data, hence preserving the credibility of scientific research.
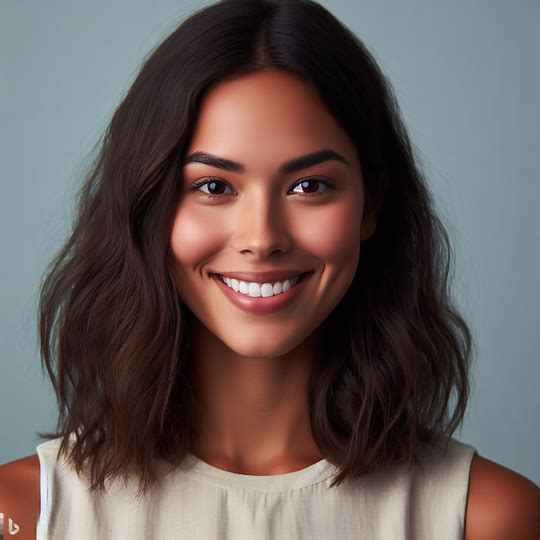
Amara Rainforest is an authoritative voice in business analysis, blending her MBA in Finance insights with real-world consulting experience. She distills complex market trends into clear, actionable advice for her readers. Her engaging writing captures the essence of modern business challenges and triumphs, making her a must-follow for aspiring entrepreneurs and seasoned executives alike.